Goals and Background
The goal of this lab is to obtain skills needed to perfomr key photogrammetric tasks on aerial photographs and satellite images. This lab is designed to understand the math behind the calculations of photographic scales, measurement of areas and perimeters of features, and calculating relief displacement. There is an introduction to stereoscopy and performing orthorecticication on satellite images.
Methods
Scales, Measurements, and Relief Displacement
The first part of this lab is calculating the scale of nearly vertical aerial photographs. Calculating the scale can be done in a couple different manners.
First, the scale was calculated measuring two points on the aerial photograph and compare that measurement to the real world distance. Though this is a great and simple method it isn't always possible to obtain a measurement in the real world.
Utilizing an image labeled with point A to point B, the measure with my ruler and got a measurement of 2.5 inches (Figure 1). The dimension in the real world from point A to point B was 8822.47 ft. The real world dimension to inches which was 105869.64. The expression to get the scale value is below:
2.5 in./8822.47 ft = 2.5/105869.64 = 1:42,348
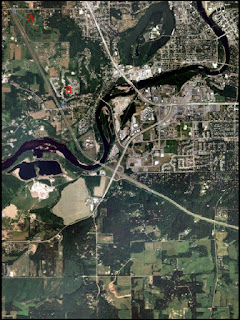 |
Figure 1. Aerial Photo of the City of Eau Claire displaying points A to B. This image is not shown to the scale the that measurements were taken. |
Photographic scale can be calculated without a true real world measurement as long as the focal length of the camera and the flying height above the terrain of the aircraft is known. The information given was as follows: Aircraft altitude= 20,000 ft, Focal length of camera=152 mm, and the elevation of the area in the photograph=769 ft. The photographic scale can be calculated using the formula:
S = F/(H-h)
S = .4986816/(20000-796)
The formula gives a scale of 1:40,000 when it is rounded to the nearest thousand.
Measurement of areas of features on aerial photographs
For this section of the assignment I will be utilizing Erdas Imagine to calculate the area of a lagoon in an aerial image (Figure 2).
By utilizing the polygon measurement tool in the measure tool bar, the area of the lagoon provided can be calculated. This is don by tracing the outline of the lagoon. From there different measurements can be changed to get multiple measurements. The results included:
- Area = 38.0736 ha and 94.0820 acres
- Perimeter = 8390.74 meters and 5.213767 miles
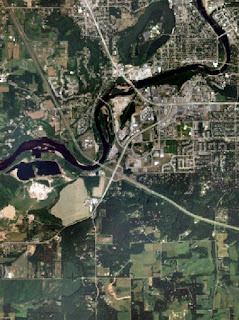 |
Figure 2. Photo used in Erdas to measure the area and perimeter of the lagoon located on the west side of the image. |
Calculating relief displacement from object height
Relief displacement is the variation of objects and features within an aerial image from their true planimetric position on the ground. Assessing Figure 3, the smoke stack (labeled 'A') is at an angle. Therefore, the smoke stack is showing displacement related to the location of the Principal Point and the location of the feature.
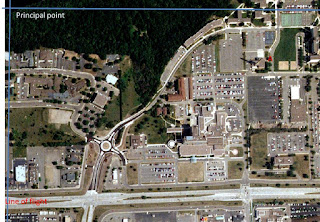 |
Figure 3. Aerial photo used to show relief displacement of the smoke stack at point A. This image is not shown to the scale the that measurements were taken. |
The scale of the image is 1:3209 and the camera height is 3,980 ft. To calculate relief displacement the formula is D= hr/H.
- h = the height of the object in the real world
- r = radial distance from the principal point to the top of the displaced object
- H = the height of the camera when the image was taken
In order to complete the formula, the real world smoke stack needed to be measured from the distance from the principal point to the top of the smoke stack. Using the scale and a ruler the determined height of the smoke stack was 802.25 inches and the radial distance from the principal point was 5 inches.
802.25 in. *5 in. / 3209 ft = 802.25 in * 5 in. / 38508 in. = 0.1042
To correct this image the smoke stack would have to be pushed back the .1042 inches to make vertical.
Stereoscopy
Stereoscopy is the science of depth perception utilizing your eyes or other tools to view a 2 dimensional (2D) image in 3 dimensions (3D). The tools Stereoscope, Anaglyph and Polaroid glasses, were used to develop and view 2D images in 3D. Erdas Imagine was used to create an Anaglyph. Anaglyph tool is selected in the Terrain menu tab. An aerial image of the City of Eau Calire and a Digital Elevation Model (DEM) was used in the Anaglyph Generation menu. Then next step is to run the tool. Once this is complete, the image can be opened up in Erdas and viewed using 3D glasses to show a different way of looking at a 3D model.
Orthorectification
Orthorectification refers to simultaneously removes positional and elevation errors from one or multiple aerial photographs or satellite images. This process requires the analyst to obtain real world x,y, and z coordinates of pixels on aerial images and photographs. Orthorectified images can be utilized to create many products such as DEM's, and Stereopairs.
This section uses Erdas Imagine Lecia Photogrammetric Suite (LPS). This tool with in Erdas Imagine is used for triangulation, orthorectification with digital photogrammetry collected by varying sensors. Additionally, it can be used to extract digital surface and elevation models. Two images were provided and placed in Erdas. Because the images overlapped they needed to be corrected.
First, a New Block File was created in the Imagine Photogrammetry Project Manager window. In the Model Setup dialog window the Geometric Model Category was set to Plynomial-based Pushbroom and selected SPOT Pushbroom in the second section window. In the Block Property Setup I set the projection to UTM, the Spheroid Name to Clarke 1866, and the Datum to NAD 27 (CONUS).
The next step the point measurement tool was activated to collect GCP's. The point measurement tool was set to Classic Point Measurement Tool. Using the Reset Horizontal Reference icon, the GCP Reference Source was set to Image Layer. Then, 11 GCP's were located on the uncorrected image and the reference image (Figure 4).
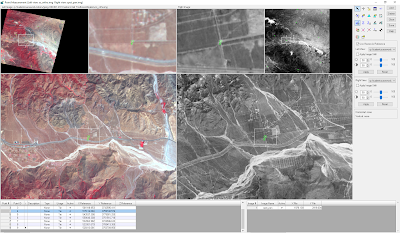 |
Figure 4. Screen shot of the Imagine Photogrammetry Project Manager window with the first 9 GCPs and their information. |
Vertical Reference Source needed to be updated and collect elevation information utilizing a DEM. Clicking on the Reset Vertical Reference Source icon opens a menu to set the Vertical Reference Source to a selected DEM. After selecting the DEM in the menu all of the values in the cell array were selected and the Z Values were updated on Selected Points icon (Figure 5).
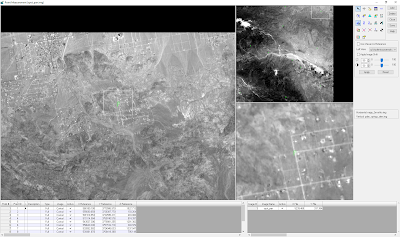 |
Figure 5. Updated GCP points after the Z values were added. |
After all of the GCP's were added and the elevation was set to the first image, the second image needed to be imported for correction. The Type and Usage had to be set for each of the control points. Type to Full and the Usage to Control for each of the GCP's. The Add Frame icon and add the second image for orthorectification was used. I set the parameters and verified the SPOT Sensor specifications the same as the first image. Only the GCP's that were within the overlap of the two images were corrected (1,2,5,6,8,9,12).
Lastly, Automatic Tie Point Generation Properties icon to calculate tie points between the two images. Tie points are points which have an unknown ground coordinates but are able to be visually identified in the overlap area of images. The coordinates of the tie points are calculated during a process called block triangulation. Block triangulation requires a minimum of 9 tie points to process.
In the Automatic Tie Point Generation Properties window set the Image used to All Available and the Initial Type to Exterior/Header/GCP. Under the distribution tab the Intended Number of Points/Images were set to 40.
Triangulation tool was ran after setting the parameters as follows:
- Iterations wtih Relaxation was set to a value of 3
- Image Coordinate Units for Report was set to Pixels.
- Type to Same as Weighted Values and the X,Y, and Z values to 15 to assure the GCP's are accurate with in 15 meters.
After running the tool a report was displayed to asses the accuracy (Figure 6).
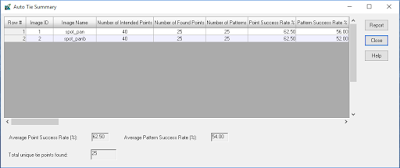 |
Figure 6. AutoTie Summary from Erdas Imagine. |
The final step including running the Ortho Resampling Process.
Results
The final results of the Orthorectification resulted in accurately positioned images (Figure 7).
 |
Figure 7. Final product of the two images after Orthorectification. |
Sources
National Agriculture Imagery Program (NAIP) images are from United States Department of
Agriculture, 2005.
Digital Elevation Model (DEM) for Eau Claire, WI is from United States Department of
Agriculture Natural Resources Conservation Service, 2010.
Lidar-derived surface model (DSM) for sections of Eau Claire and Chippewa are from Eau
Claire County and Chippewa County governments respectively.
Spot satellite images are from Erdas Imagine, 2009.
Digital elevation model (DEM) for Palm Spring, CA is from Erdas Imagine, 2009.
National Aerial Photography Program (NAPP) 2 meter images are from Erdas Imagine, 2009.